February 9, 2024
AI solution avoids weather-dependent fluctuations in the power system
FIAS researchers develop technology to stabilise the use of renewable energies in the grid.
The high amount of solar and wind energy makes it difficult to ensure a stable power supply. A state-of-the-art AI system developed by FIAS researchers compensates for these weather-dependent fluctuations. It ensures efficient and reliable distribution of electricity from sources such as solar and wind to meet energy needs even in changing weather conditions while minimising costs.
In a breakthrough study, PhD student Chen Li, led by FIAS Fellow Kai Zhou, presents a new AI technology that could revolutionise the incorporation of renewable energy into the power grid. Integrating renewable energy sources such as wind and solar power is not easy. Changes in the weather have a rapid impact on power generation and require frequent adjustments to the power grid. For example, even a single cloud over a photovoltaic system can cause major fluctuations in electricity within a very short space of time. Traditional methods face difficulties in dealing with this variability, especially in large power systems. The FIAS research team developed a cutting-edge, physics-informed machine learning method that can quickly adapt to changing conditions, making it ideal for real-time applications.
Based on electricity demand and weather patterns, the team's approach offers a solution for the immediate equalisation of fluctuations. It is based on innovative neural networks based on graphical data on weather and power demand, the graph attention networks. It identifies the key nodes in the power grid, that mainly influence the pattern of power dispatch. The method also improves transparency in AI decision-making and provides insights how neural networks interpret data to make optimal decisions on energy distribution.
The current publication demonstrates the superiority of this method over existing data-driven techniques in two different scale scenarios for renewable energy systems. The new approach not only provides prompt and feasible solutions, but also maintains interpretability, which is crucial for understanding and trusting AI-based systems.
This technology is still at an early stage. It has mainly been tested on small and medium-sized power grids. "But it will change modern energy systems," Chen Li is convinced: "It enables the seamless integration of distributed energy sources into the grid, primarily solar cells and wind turbines". This will make renewable energies more reliable and easier to use. The system improves the efficiency, reliability and sustainability of electricity distribution and enables intelligent management and regular adjustment of the electricity grid. It also lays the groundwork for economic optimisation by considering a comprehensive range of physical factors and the dynamics of the energy market.
"As we continue to explore and improve this technology, we move closer to a cleaner, greener, and more sustainable future," says physicist Li. The team wants to further develop the system to include energy storage solutions with the help of AI.
Publication: Chen Li, Alexander Kies, Kai Zhou, Markus Schlott, Omar El Sayed, Mariia Bilousova, Horst Stoecker, Optimal Power Flow in a Highly Renewable Power System Based on Attention Neural Networks, Applied Energy 359 (2024) 122779, https://doi.org/10.1016/j.apenergy.2024.122779
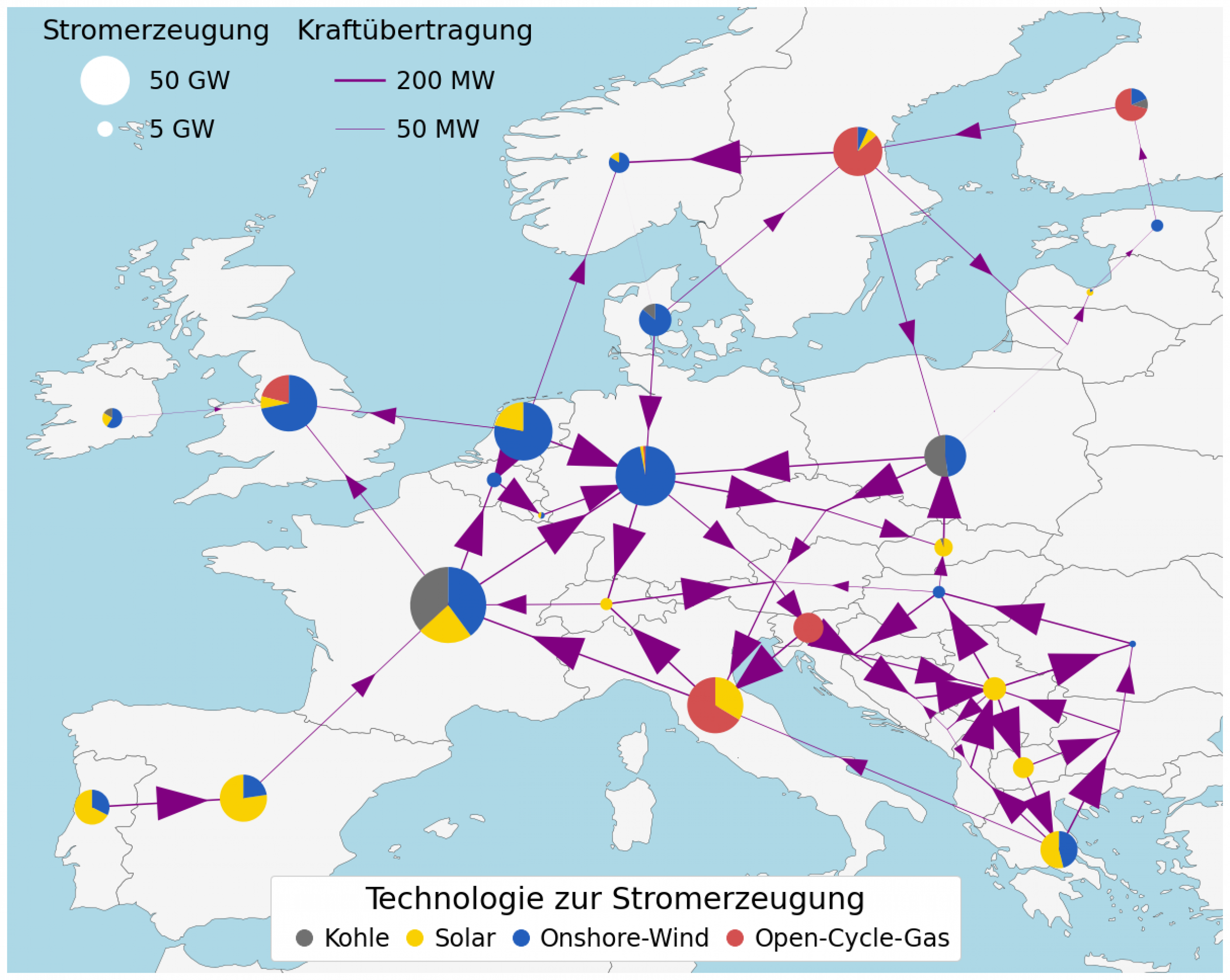