October 6, 2022
Improved early earthquake warning with Artificial Intelligence
A significantly improved model for earlier earthquake detection is described in two recent publications of Nishtha Srivastava’s group at the Frankfurt Institute for Advanced Studies (FIAS). Their model detects earthquakes more reliably and faster than previous studies, which can simplify advance warnings, especially in less developed regions of the world.
The input for the deep learning based model developed by the team of Srivastava are oscillations that are transmitted particularly faster through the earth's layers as compared to following more devastating waves. These primary(P)-waves precede the stronger tremors - by seconds or minutes, depending on the distance from the source of the quake. "This short time lead may provide enough time window for civilians to get to safety," Srivastava points out. The following secondary(S)- and surface waves are of higher amplitude, more dangerous and cause greater damage.
The Convolutional Recurrent Model for Earthquake Identification and Magnitude Estimation - CREIME, developed by the scientists, performs three tasks: It discriminates the quake against background noise, detects the P-wave arrival and even estimates the strength of the following quake. "Traditionally, a network of measuring stations has been necessary for a reliable prediction," explains Megha Chakraborty (25), FIAS PhD student and first author of both publications. "With our model, one station is sufficient to issue a warning for earthquakes within a distance of approximately 350 kilometres." Existing methods often require extensive human involvement and empirical relations. They are also very sensitive to background noise. CREIME differentiates between seismic events and noise with an average accuracy of 98 percent.
CREIME estimates the earthquake magnitude within two seconds of the arrival of the first P-wave. The authors demonstrate this with two independent data sets from Italy and worldwide. "Our prediction accuracy can outperform classical methods by detecting up to 10 percent more events", says Srivastava. With a deep-learning approach, the system is also constantly improving: PolarCAP, another deep learning model that uses convolutional autoencoders, can automatically identify the vertical direction of first motion during an earthquake which is important in quantifying its underlying fault mechanism.
The team now wants to test the CREIME and PolarCAP prototype in real time settings. "In regions like Southern California or Indonesia, where the epicenters can be very close to human settlements, an app based on our model could in future warn people early enough for them to find shelter," Chakraborty hopes.
Publications:
- Chakraborty, M., Fenner, D., Li, W., Faber, J., Zhou, K., Rümpker, G., et al. (2022). CREIME—A Convolutional Recurrent model for Earthquake Identification and Magnitude Estimation. Journal of Geophysical Research: Solid Earth, 127, e2022JB024595. https://doi.org/10.1029/2022JB024595
- Megha Chakraborty, Claudia Quinteros Cartaya, Wei Li, Johannes Faber, Georg Rümpker, Horst Stoecker, Nishtha Srivastava, PolarCAP – A deep learning approach for first motion polarity classification of earthquake waveforms, Artificial Intelligence in Geosciences (2022) 3, 46-52, ISSN 2666-5441, https://doi.org/10.1016/j.aiig.2022.08.001
Image/GIF: The CREIME Model processes 5 second windows to detect an earthquake. It can issue warnings within second of P-wave arrival, so people at risk can take shelter in time before dangerous S-/surface waves arrive. Click here for GIF by Megha Chakraborty, FIAS
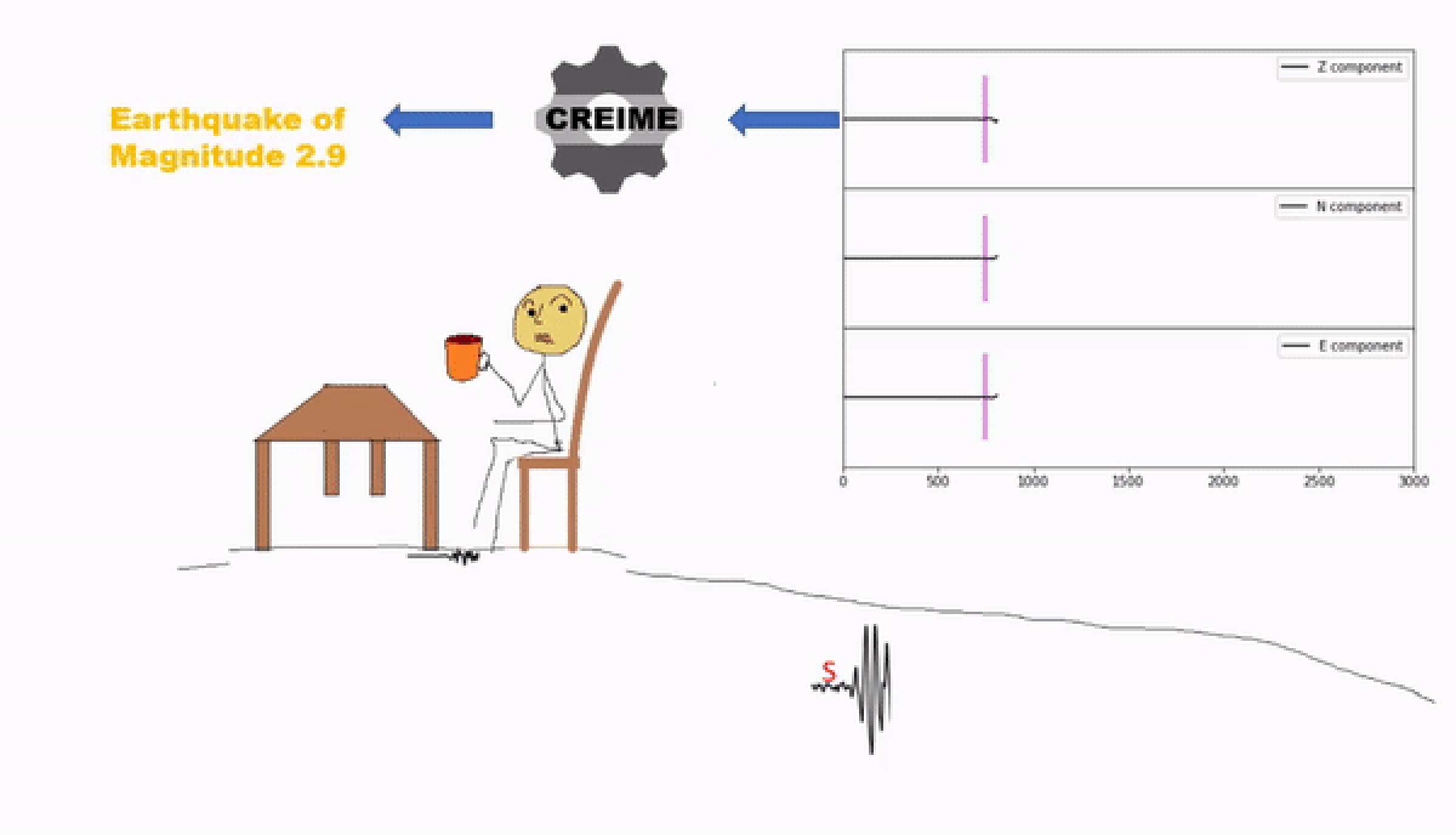