May 30, 2022
Measuring volcanic activity
The FIAS Seismology and Artificial Intelligence group used the Italian volcano Stromboli in the Mediterranean Sea as an example to develop a powerful module for immediately detecting and creating more complete seismo-volcanic event catalogs. These catalogs not only enable the evaluation of volcanic hazard but also the in-depth investigation of possible precursors based on the historic instrumental data.
To understand and predict volcanic activity, data on its activities are collected worldwide. This is particularly complicated for Strombolian volcanoes, which are characterized by frequent mild eruptions. However, dangerous major eruptions can occur. For instance, Stromboli last erupted violently in 2019; resulting in a kilometer high ash-gas plume and one fatality.
For the detailed analysis of these volcanoes, labeled datasets with information about seismo-volcanic events are required, especially for the application of deep learning methods. Therefore, volcanoes are monitored and analysed worldwide; nonetheless, this requires a lot of manual work.
To automatically analyse seismic data and identify events in this study, Darius Fenner (20) from Nishtha Srivastava's FIAS research team in collaboration with Georg Rümpker and Horst Stöcker developed the Adaptive-Window Volcanic Event Selection Analysis Module - AWESAM. This algorithm uses unlabeled raw seismic waveforms to extract time and amplitude information. A special filter allows to register both rare violent and regular small events. In a second step, these measurements are matched with another station to exclude local interfering factors. The strength of this method is the reliable detection of very small and frequent events as well as major explosions.
The scientists used publicly accessible continuous seismic recordings from two almost equidistant stations at Stromboli volcano in Italy as an example. The module allows for a straightforward generalization and application to other volcanoes with frequent Strombolian activity worldwide. Furthermore, this module can be implemented for volcanoes with rarer explosions.
This research was executed by Darius Fenner, a first-year Physics Bachelor student at Johannes Gutenberg University-Mainz (JGU), along with the ‘Seismology and Artificial Intelligence -SAI’ research team. SAI research team lead by Nishtha Srivastava is funded by the German Federal Ministry of Education and Research (BmBF) with more than 1.6 million Euros as part of the "Promotion of female AI junior scientists" program. SAI group seeks to apply Deep Learning and Machine Learning based algorithms on seismic signals to improve Earthquake Early Warning System (EEWs) and Seismic Signal Analysis. Additionally, they are also trying to better understand the pattern associated with Seismic stress release
Publication:
Darius Fenner, Georg Rümpker, Wei Li, Megha Chakraborty, Johannes Faber, Jonas Köhler, Horst Stöcker and Nishtha Srivastava, Automated Seismo-Volcanic Event Detection Applied to Stromboli (Italy), Front. Earth Sci. 10:809037, doi: 10.3389/feart.2022.809037, https://www.frontiersin.org/articles/10.3389/feart.2022.809037/full
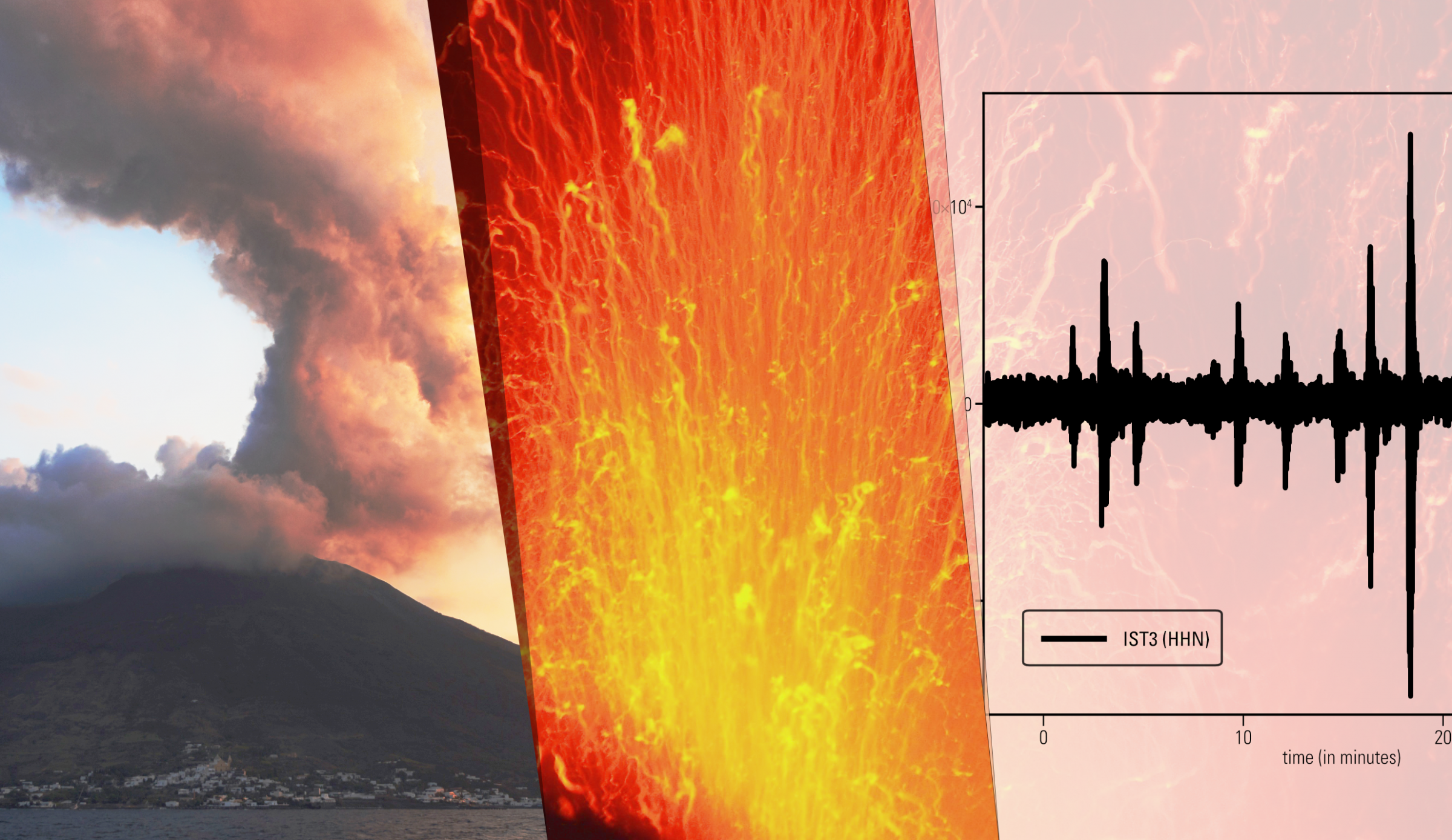