March 22, 2023
Algorithms illuminate lightning structures
Lightning is a natural phenomenon that has fascinated humans for centuries. However, understanding the complex structures of lightning has been a challenge for scientists due to the vast amount of data involved. In a recent study, researchers from FIAS and University of Groningen have developed a novel method to understand lightning structures using machine learning. This knowledge will also help us to avoid lightning dangers in the future.
Analyzing lightning data can be very time-consuming and so far depends on photos for studying the structure of lightning. A single lightning flash contain hundreds of some fine and complex structures and a myriad of different phenomena. To address this challenge, Lingxiao Wang and his colleagues Brian Hare, Horst Stöcker, Kai Zhou, and Olaf Scholten have developed a novel method to identify structures in lightning data using machine learning algorithms and correlation analysis. They used data from the Dutch-German radio telescope LOFAR (Low Frequency Array), a network of many individual antennas. “The exploration of the lightning structure is the first step to understand the occurrence and evolution of lightning phenomena”, Wang explains. “If we understand such extreme phenomena better in the near future, it will help us to avoid lightning disasters”.
The study, published in the Chaos, Solitons and Fractals journal, sheds light on the understanding of lightning structures and provides insights into the complicated correlation functions for different lightning phenomena. The use of machine learning algorithms can help identify structures from numerous spatio-temporal points in a high dimensional space, which is very time-consuming when done by-eye. This novel method is a powerful tool to search vast multidimensional data sets for unique structures.
The findings of this study have potential applications beyond the field of physics. The combination of algorithms used in this study (t-distributed stochastic neighbor embedding, t-SNE and a clustering algorithm) can be applied to search vast multidimensional data sets for unique structures in other fields beyond physics – “for example data of biological or medical methods, earthquakes, or human mobility”, Wang says.
This research highlights the FIAS's commitment to advancing scientific knowledge through innovative research methods. By developing new tools and techniques to analyze complex data sets, our researchers are making significant contributions to our understanding of natural phenomena.
Publications:
Lingxiao Wang, Brian M. Hare, Kai Zhou, Horst Stöcker, and Olaf Scholten, Identifying Lightning Structures via Machine Learning, Chaos, Solitons & Fractals 170, 113346 (2023).
Brian M Hare, Olaf Scholten, Joseph Dwyer et al. Needle-like structures discovered on positively charged lightning branches, Nature, 2019, 568(7752): 360-363.
Find a short feature in German TV ("Alle Wetter", Hessischer Rundfunk) here.
Figure: Electrical charge in the air creates a small pathway of charged air from the storm cloud towards the ground, the “leader”. A newly discovered phenomenon are small, sharp pathways of charged air, the "needles". Lightning data from radio telescopes can be used to calculate their fine structure by artificial intelligence. The photo was also generated by Artificial Intelligence (Lingxiao Wang).
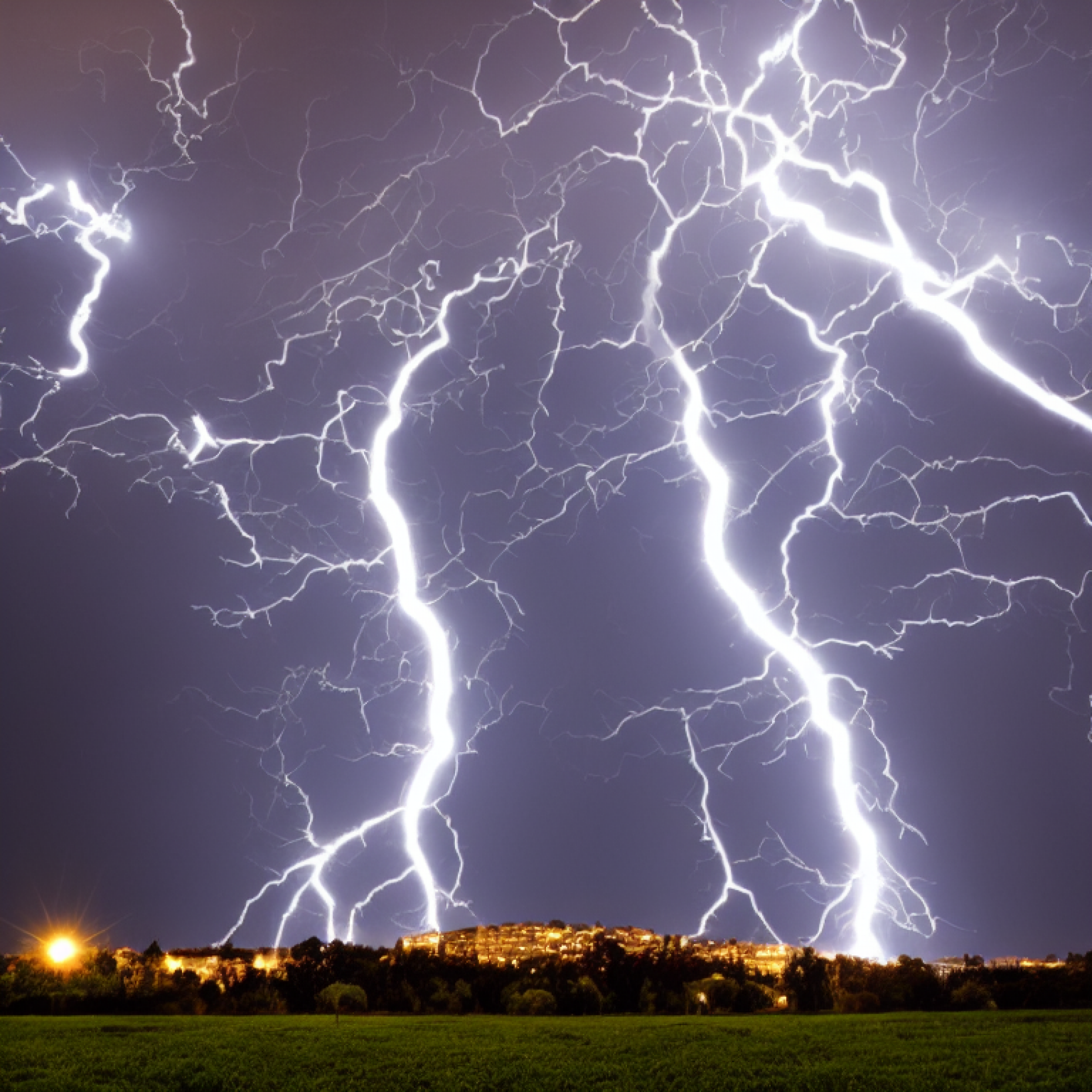