March 9, 2023
KISS: FIAS part of joint project for AI simulation of scientific data
KISS enables faster, more flexible and more efficient evaluation of research data based on AI simulations. With Dr. Kai Zhou as PI, FIAS is participating in the joint project "Artificial Intelligence for the Fast Simulation of Scientific Data" (KISS) funded by the Federal Ministry of Education and Research (BMBF).
Simulations play a key role in scientific research - from fundamental physics research to application areas such as biology, robotics, or climate modelling. Particularly in high-energy nuclear physics in the area of "Exploration of Universe and Matter" (ErUM), simulations are necessary starting from a detailed mathematical understanding of fundamental physical processes. This is the only way to achieve the physical goals of big infrastructures such as high-energy accelerators, which are particularly computationally intensive, resource-intensive and time-consuming. With the expansion of detectors and telescopes, computing capacities are reaching their limits. Thus, increase in the efficiency of the related simulation algorithms is crucial for the successful operation of these large high-energy experiments. The KISS project aims at AI-based simulation processes that enable a faster, more flexible and more efficient evaluation of research data than conventional methods.
As part of the "ErUM-Data" action plan, the BMBF is funding Zhou’s research with 240.000 Euros for the next three years. In addition to Kai Zhou's working group, teams from Hamburg, Dortmund, Dresden, Göttingen, Heidelberg and Munich are involved. As part of KISS, the FIAS team will develop generative AI models that replace and accelerate classical detailed modelling of heavy ion collisions. This includes, for example, the high-resolution detector simulations (Geant4) for experiments such as "Compressed Baryonic Matter" (CBM) at GSI/FAIR or "A large Ion collider Experiment" (ALICE) at CERN. Zhou's group researches modern generative architectures such as Generative Adversarial Networks (GANs), Variational Autoencoders (VAEs), Normalising Flows (NFs) and Diffusion Models (DMs) and creates the respective simulation task. These developments can largely mitigate the huge computational and memory demand in the physical exploration and data acquisition phases of experiments.
Such processes can also be applied to other important needs of society and industry, such as climate modelling and prediction, robotics simulations, 3D prototype design in industry. Two already successful and popular general applications for this kind of generative AI models are ChatGPT and DALL-E2.
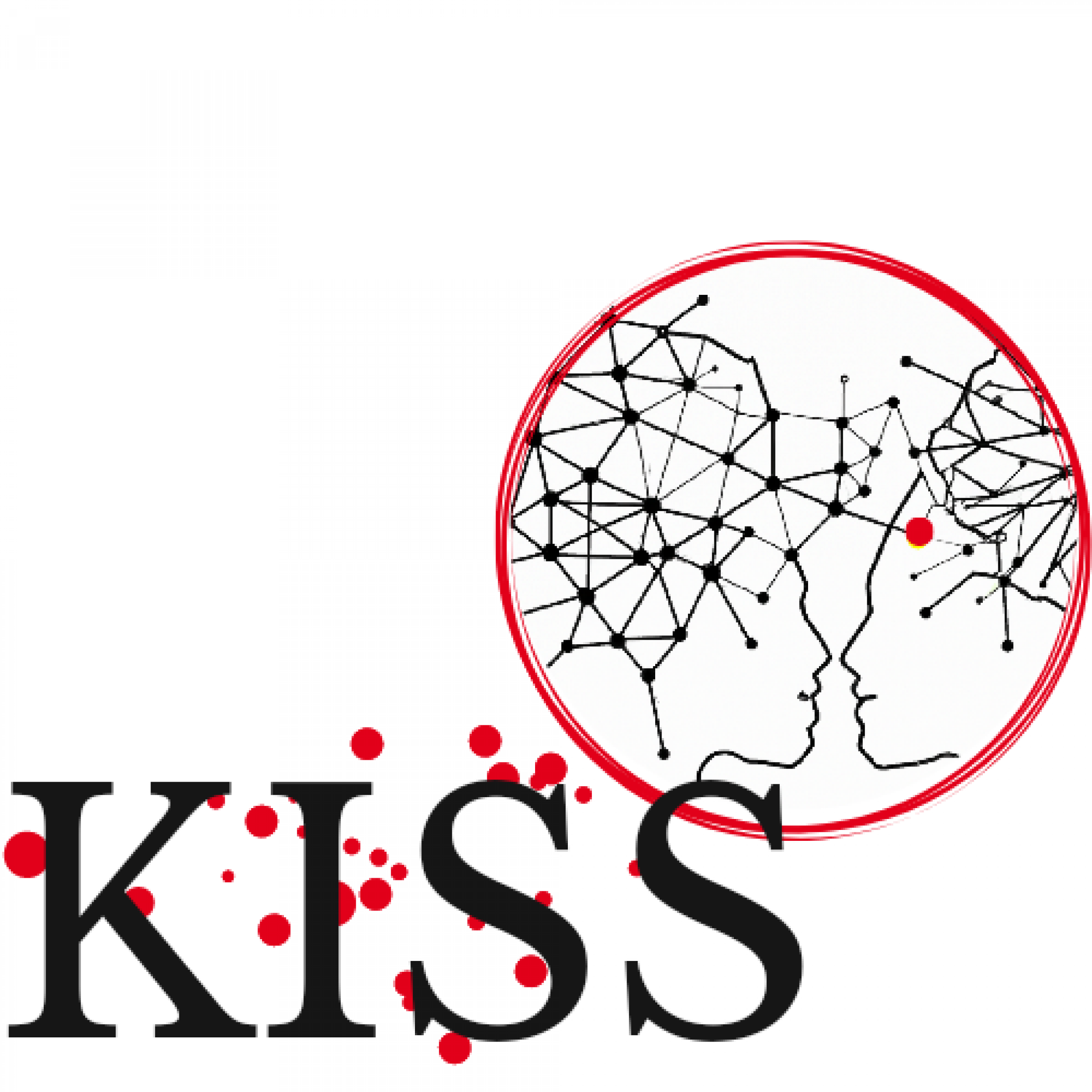