November 25, 2020
Deep learning in heavy ion physics
New models allow faster analysis of collision events
In order to make better use of the amount of data generated by high-energy experiments, scientists at FIAS have developed a new Deep Learning (DL) analysis method. The models based on the PointNet architecture enable a fast online analysis of collision events at event rates of several million collisions per second. For this purpose, the determination of the centrality of heavy ion collisions at the Compressed Baryonic Matter Experiment (CBM) of the FAIR facility, which is currently under construction at the GSI Helmoltzzentrum für Schwerionenforschung in Darmstadt, was exemplarily investigated.
The CBM experiment is designed to answer one of the central questions in physics, the generation of the mass of atomic nuclei. There are many theories on this subject, but there is no comprehensive experimental confirmation for this yet. With the Facility for Antiproton and Ion Research (FAIR) in Darmstadt, a new accelerator facility is currently being built with the aim, among other things, of answering this question. This is also where the CBM experiment is being built, which will study the properties of hadrons (e.g. neutrons and protons) in an environment that corresponds to many times the density of atomic nuclei. Such highly compressed nuclear matter is only created in high-energy collisions of ionized nuclei and exists only for tiny fractions of a second. The elementary particles generated from these collisions can be detected and studied with the CBM detector. A unique feature of the new CBM experiment is that several million such collision events per second can be generated and recorded. This extremely large data stream requires ultra-fast real-time analysis. This requires completely new analysis methods. FIAS scientists have now developed a new method for event characterization based on deep learning methods. This new method of event classification proves to be more accurate and less model-dependent than conventional methods and uses state-of-the-art GPU processor units to improve performance.
The PointNet models used by Manjunath Omana Kuttan and his colleagues are based on a deep learning architecture optimized for learning from point cloud data. Point clouds are collections of disordered points in space, where each point represents the multi-dimensional properties of an element that contributes to the cloud's collective structure. In the case of a heavy ion collision, for example, these points correspond to the properties of particle tracks in the CBM detector.
For their study, the researchers used the UrQMD model, also co-developed at FIAS, and the detector simulation of CBM to generate gold-gold collision events at an energy of 10 AGeV. These could then be used to train the PointNet-based architectures. The models were trained using features such as the trajectories of particles in the CBM detector planes. In the future it should be possible to use the developed model architectures in other heavy ion collision experiments, e.g.: ALICE at the Large Hadron Collider (LHC) or HADES at GSI's SIS18. The researchers hope to generalize the developed deep learning models for the analysis of a variety of different properties of the nuclear collisions.
Further Information:
Original publication:
Manjunath Omana Kuttan, Jan Steinheimer, Kai Zhou, Andreas Redelbach, Horst Stoecker,
"A fast centrality-meter for heavy-ion collisions at the CBM experiment", Physics Letters B, Volume 811, 2020, 135872, ISSN 0370-2693, https://doi.org/10.1016/j.physletb.2020.135872
Picture:
Visualization of a point cloud of all hits in the STS detector of the CBM experiment.
Source: Simulations by Manjunath Omana Kuttan Background 3D model of the CBM experiment: doi: 10.1140/epja/i2017-12248-y
More about the CBM experiment: https://www.cbm.gsi.de
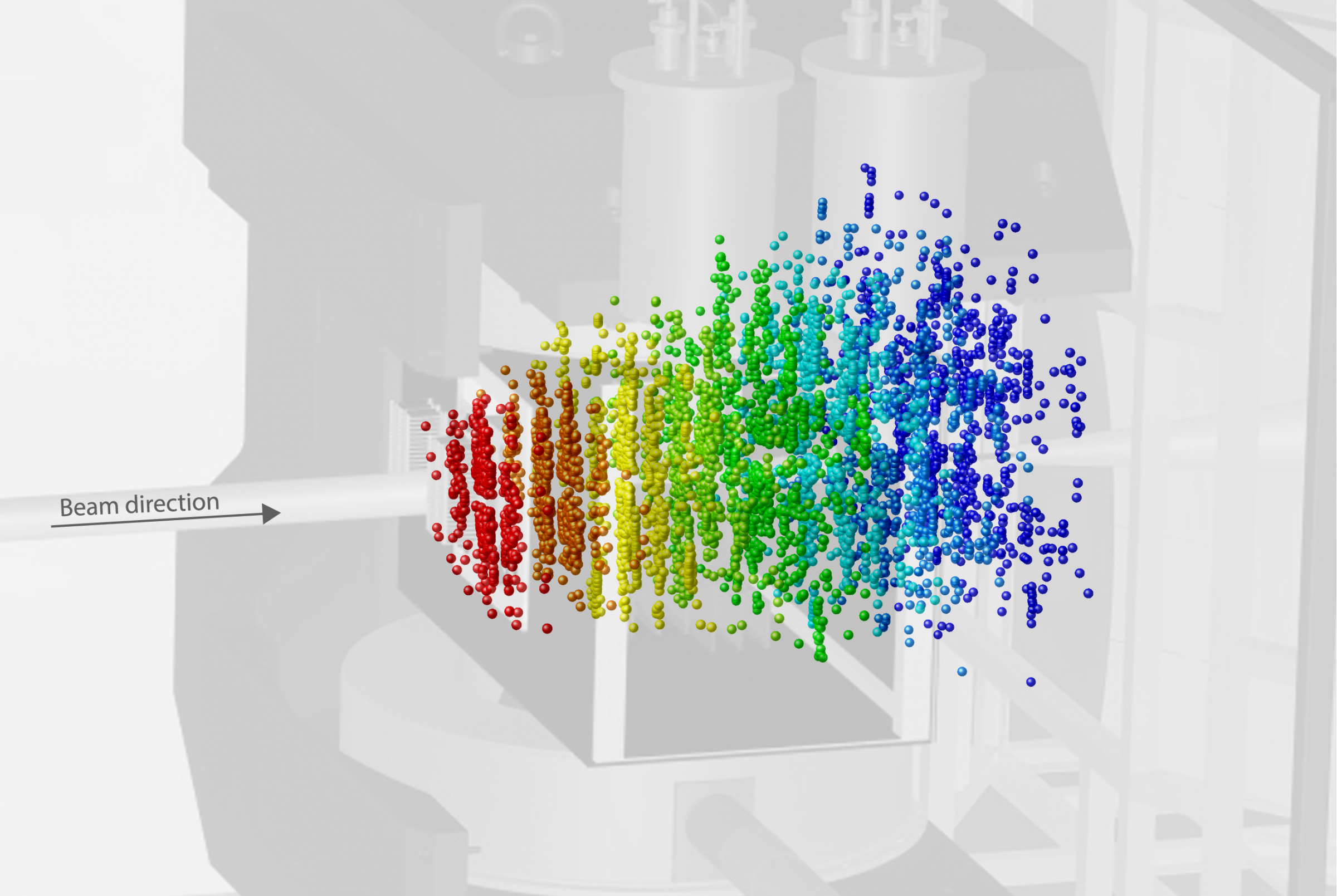