October 9, 2024
New AI model prevents damage in industrial pipes
FIAS research team achieves high accuracy in cavitation detection
Vapour bubbles are feared in industrial plants. They form in pipes when pressurised liquid begins to boil or vaporise. The bubbles that form and suddenly collapse damage pumps and equipment. A research group at FIAS is now presenting a new approach to detecting these ‘cavitations’, which could significantly improve industrial pipework management. All industries with fluid systems such as water, energy and manufacturing could benefit.
Under the leadership of FIAS Fellow Kai Zhou, PhD student Yu Sha presents a Sub-Master Transition Network (SMTNet) in a recent study in the renowned journal ‘Expert Systems with Applications’. This innovative deep learning model overcomes the critical limitations of current methods for recognising such cavitations. The vapour bubbles that form and collapse in liquids can cause considerable damage to industrial plants. The approach developed by SMTNet provides a nuanced and accurate method for detecting cavitation intensity, potentially saving industry millions in maintenance costs and downtime.
Among SMTNet's key innovations is a multi-stage mechanism that mimics expert cavitation detection while enabling more intuitive and accurate classifications. In addition, a filter stage signals long-term changes with acoustic signals and detects subtle patterns that other models miss. A double hierarchical 1D residual block detects sensitive features acoustically. In addition, a hierarchial multi-label tree maps the structure of cavitation states, providing more informative and interpretable results.
The SMTNet model was thoroughly tested on three real cavitation data sets from the valve technology manufacturer SAMSON AG in Frankfurt and showed remarkable performance. It achieved an accuracy for cavitation of up to 100 %, clearly outperforming existing methods.
The approach represents a paradigm shift in the detection and classification of cavitation events. The research group utilised the hierarchical nature of cavitation states to develop a model that not only outperforms current methods, but also provides results that are more in line with the perceptions of experts in the field of cavitation. This breakthrough has far-reaching implications for industries that rely on fluid systems, including water management, power generation and manufacturing. The improved accuracy and interpretability of the SMTNet model promises to improve maintenance strategies, reduce equipment failures and improve overall system efficiency.
‘There is still room for improvement, especially in recognising the onset of cavitation in some scenarios,’ admits Zhou. ‘Our future research will focus on further refining the model and developing a one-step framework for cavitation intensity detection.’ The current study is already a significant step forward, he adds.
Publication: Shuiping Gou, Yu Sha, Bo Liu, Ningtao Liu, Johannes Fabe, Stefan Schramm, Horst Stoecker, Thomas Steckenreiter, Domagoj Vnucec, Nadine Wetzstein, Andreas Widl, Kai Zhou, Hierarchical cavitation intensity recognition using Sub-Master Transition Network-based acoustic signals in pipeline systems, Expert Systems with Applications, Volume 258, 2024, 125155, ISSN 0957-4174, https://doi.org/10.1016/j.eswa.2024.125155.
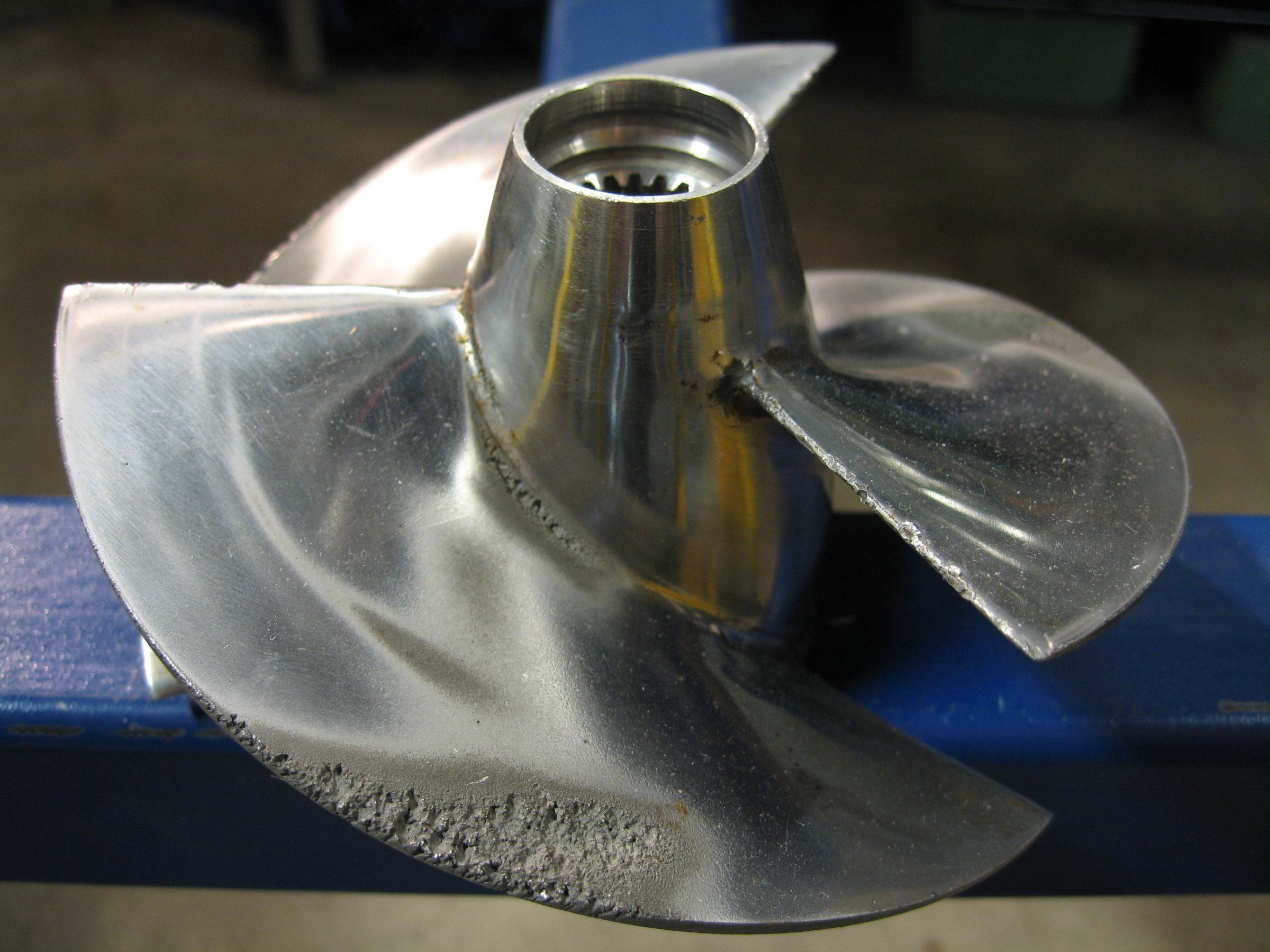